Kubernetes News
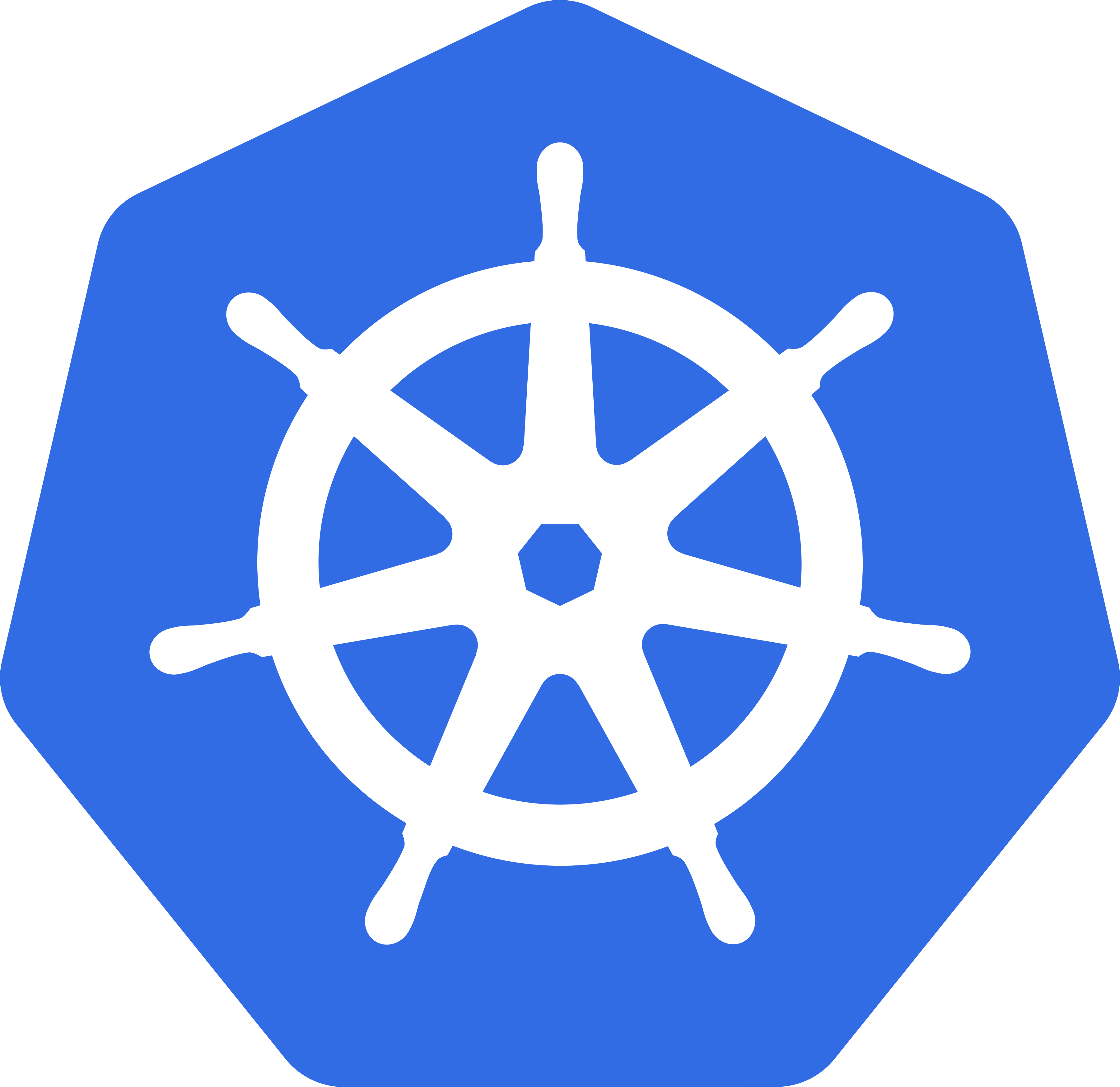
-
Spotlight on Kubernetes Upstream Training in Japan
About our team
We are organizers of Kubernetes Upstream Training in Japan. Our team is composed of members who actively contribute to Kubernetes, including individuals who hold roles such as member, reviewer, approver, and chair.
Our goal is to increase the number of Kubernetes contributors and foster the growth of the community. While Kubernetes community is friendly and collaborative, newcomers may find the first step of contributing to be a bit challenging. Our training program aims to lower that barrier and create an environment where even beginners can participate smoothly.
What is Kubernetes upstream training in Japan?
Our training started in 2019 and is held 1 to 2 times a year. Initially, Kubernetes Upstream Training was conducted as a co-located event of KubeCon (Kubernetes Contributor Summit), but we launched Kubernetes Upstream Training in Japan with the aim of increasing Japanese contributors by hosting a similar event in Japan.
Before the pandemic, the training was held in person, but since 2020, it has been conducted online. The training offers the following content for those who have not yet contributed to Kubernetes:
- Introduction to Kubernetes community
- Overview of Kubernetes codebase and how to create your first PR
- Tips and encouragement to lower participation barriers, such as language
- How to set up the development environment
- Hands-on session using kubernetes-sigs/contributor-playground
At the beginning of the program, we explain why contributing to Kubernetes is important and who can contribute. We emphasize that contributing to Kubernetes allows you to make a global impact and that Kubernetes community is looking forward to your contributions!
We also explain Kubernetes community, SIGs, and Working Groups. Next, we explain the roles and responsibilities of Member, Reviewer, Approver, Tech Lead, and Chair. Additionally, we introduce the communication tools we primarily use, such as Slack, GitHub, and mailing lists. Some Japanese speakers may feel that communicating in English is a barrier. Additionally, those who are new to the community need to understand where and how communication takes place. We emphasize the importance of taking that first step, which is the most important aspect we focus on in our training!
We then go over the structure of Kubernetes codebase, the main repositories, how to create a PR, and the CI/CD process using Prow. We explain in detail the process from creating a PR to getting it merged.
After several lectures, participants get to experience hands-on work using kubernetes-sigs/contributor-playground, where they can create a simple PR. The goal is for participants to get a feel for the process of contributing to Kubernetes.
At the end of the program, we also provide a detailed explanation of setting up the development environment for contributing to the
kubernetes/kubernetes
repository, including building code locally, running tests efficiently, and setting up clusters.Interview with participants
We conducted interviews with those who participated in our training program. We asked them about their reasons for joining, their impressions, and their future goals.
Keita Mochizuki (NTT DATA Group Corporation)
Keita Mochizuki is a contributor who consistently contributes to Kubernetes and related projects. Keita is also a professional in container security and has recently published a book. Additionally, he has made available a Roadmap for New Contributors, which is highly beneficial for those new to contributing.
Junya: Why did you decide to participate in Kubernetes Upstream Training?
Keita: Actually, I participated twice, in 2020 and 2022. In 2020, I had just started learning about Kubernetes and wanted to try getting involved in activities outside of work, so I signed up after seeing the event on Twitter by chance. However, I didn't have much knowledge at the time, and contributing to OSS felt like something beyond my reach. As a result, my understanding after the training was shallow, and I left with more of a "hmm, okay" feeling.
In 2022, I participated again when I was at a stage where I was seriously considering starting contributions. This time, I did prior research and was able to resolve my questions during the lectures, making it a very productive experience.
Junya: How did you feel after participating?
Keita: I felt that the significance of this training greatly depends on the participant's mindset. The training itself consists of general explanations and simple hands-on exercises, but it doesn't mean that attending the training will immediately lead to contributions.
Junya: What is your purpose for contributing?
Keita: My initial motivation was to "gain a deep understanding of Kubernetes and build a track record," meaning "contributing itself was the goal." Nowadays, I also contribute to address bugs or constraints I discover during my work. Additionally, through contributing, I've become less hesitant to analyze undocumented features directly from the source code.
Junya: What has been challenging about contributing?
Keita: The most difficult part was taking the first step. Contributing to OSS requires a certain level of knowledge, and leveraging resources like this training and support from others was essential. One phrase that stuck with me was, "Once you take the first step, it becomes easier to move forward." Also, in terms of continuing contributions as part of my job, the most challenging aspect is presenting the outcomes as achievements. To keep contributing over time, it's important to align it with business goals and strategies, but upstream contributions don't always lead to immediate results that can be directly tied to performance. Therefore, it's crucial to ensure mutual understanding with managers and gain their support.
Junya: What are your future goals?
Keita: My goal is to contribute to areas with a larger impact. So far, I've mainly contributed by fixing smaller bugs as my primary focus was building a track record, but moving forward, I'd like to challenge myself with contributions that have a greater impact on Kubernetes users or that address issues related to my work. Recently, I've also been working on reflecting the changes I've made to the codebase into the official documentation, and I see this as a step toward achieving my goals.
Junya: Thank you very much!
Yoshiki Fujikane (CyberAgent, Inc.)
Yoshiki Fujikane is one of the maintainers of PipeCD, a CNCF Sandbox project. In addition to developing new features for Kubernetes support in PipeCD, Yoshiki actively participates in community management and speaks at various technical conferences.
Junya: Why did you decide to participate in the Kubernetes Upstream Training?
Yoshiki: At the time I participated, I was still a student. I had only briefly worked with EKS, but I thought Kubernetes seemed complex yet cool, and I was casually interested in it. Back then, OSS felt like something out of reach, and upstream development for Kubernetes seemed incredibly daunting. While I had always been interested in OSS, I didn't know where to start. It was during this time that I learned about the Kubernetes Upstream Training and decided to take the challenge of contributing to Kubernetes.
Junya: What were your impressions after participating?
Yoshiki: I found it extremely valuable as a way to understand what it's like to be part of an OSS community. At the time, my English skills weren't very strong, so accessing primary sources of information felt like a big hurdle for me. Kubernetes is a very large project, and I didn't have a clear understanding of the overall structure, let alone what was necessary for contributing. The upstream training provided a Japanese explanation of the community structure and allowed me to gain hands-on experience with actual contributions. Thanks to the guidance I received, I was able to learn how to approach primary sources and use them as entry points for further investigation, which was incredibly helpful. This experience made me realize the importance of organizing and reviewing primary sources, and now I often dive into GitHub issues and documentation when something piques my interest. As a result, while I am no longer contributing to Kubernetes itself, the experience has been a great foundation for contributing to other projects.
Junya: What areas are you currently contributing to, and what are the other projects you're involved in?
Yoshiki: Right now, I'm no longer working with Kubernetes, but instead, I'm a maintainer of PipeCD, a CNCF Sandbox project. PipeCD is a CD tool that supports GitOps-style deployments for various application platforms. The tool originally started as an internal project at CyberAgent. With different teams adopting different platforms, PipeCD was developed to provide a unified CD platform with a consistent user experience. Currently, it supports Kubernetes, AWS ECS, Lambda, Cloud Run, and Terraform.
Junya: What role do you play within the PipeCD team?
Yoshiki: I work full-time on improving and developing Kubernetes-related features within the team. Since we provide PipeCD as a SaaS internally, my main focus is on adding new features and improving existing ones as part of that support. In addition to code contributions, I also contribute by giving talks at various events and managing community meetings to help grow the PipeCD community.
Junya: Could you explain what kind of improvements or developments you are working on with regards to Kubernetes?
Yoshiki: PipeCD supports GitOps and Progressive Delivery for Kubernetes, so I'm involved in the development of those features. Recently, I've been working on features that streamline deployments across multiple clusters.
Junya: Have you encountered any challenges while contributing to OSS?
Yoshiki: One challenge is developing features that maintain generality while meeting user use cases. When we receive feature requests while operating the internal SaaS, we first consider adding features to solve those issues. At the same time, we want PipeCD to be used by a broader audience as an OSS tool. So, I always think about whether a feature designed for one use case could be applied to another, ensuring the software remains flexible and widely usable.
Junya: What are your goals moving forward?
Yoshiki: I want to focus on expanding PipeCD's functionality. Currently, we are developing PipeCD under the slogan "One CD for All." As I mentioned earlier, it supports Kubernetes, AWS ECS, Lambda, Cloud Run, and Terraform, but there are many other platforms out there, and new platforms may emerge in the future. For this reason, we are currently developing a plugin system that will allow users to extend PipeCD on their own, and I want to push this effort forward. I'm also working on features for multi-cluster deployments in Kubernetes, and I aim to continue making impactful contributions.
Junya: Thank you very much!
Future of Kubernetes upstream training
We plan to continue hosting Kubernetes Upstream Training in Japan and look forward to welcoming many new contributors. Our next session is scheduled to take place at the end of November during CloudNative Days Winter 2024.
Moreover, our goal is to expand these training programs not only in Japan but also around the world. Kubernetes celebrated its 10th anniversary this year, and for the community to become even more active, it's crucial for people across the globe to continue contributing. While Upstream Training is already held in several regions, we aim to bring it to even more places.
We hope that as more people join Kubernetes community and contribute, our community will become even more vibrant!
-
Announcing the 2024 Steering Committee Election Results
The 2024 Steering Committee Election is now complete. The Kubernetes Steering Committee consists of 7 seats, 3 of which were up for election in 2024. Incoming committee members serve a term of 2 years, and all members are elected by the Kubernetes Community.
This community body is significant since it oversees the governance of the entire Kubernetes project. With that great power comes great responsibility. You can learn more about the steering committee’s role in their charter.
Thank you to everyone who voted in the election; your participation helps support the community’s continued health and success.
Results
Congratulations to the elected committee members whose two year terms begin immediately (listed in alphabetical order by GitHub handle):
- Antonio Ojea (@aojea), Google
- Benjamin Elder (@BenTheElder), Google
- Sascha Grunert (@saschagrunert), Red Hat
They join continuing members:
- Stephen Augustus (@justaugustus), Cisco
- Paco Xu 徐俊杰 (@pacoxu), DaoCloud
- Patrick Ohly (@pohly), Intel
- Maciej Szulik (@soltysh), Defense Unicorns
Benjamin Elder is a returning Steering Committee Member.
Big thanks!
Thank you and congratulations on a successful election to this round’s election officers:
- Bridget Kromhout (@bridgetkromhout)
- Christoph Blecker (@cblecker)
- Priyanka Saggu (@Priyankasaggu11929)
Thanks to the Emeritus Steering Committee Members. Your service is appreciated by the community:
- Bob Killen (@mrbobbytables)
- Nabarun Pal (@palnabarun)
And thank you to all the candidates who came forward to run for election.
Get involved with the Steering Committee
This governing body, like all of Kubernetes, is open to all. You can follow along with Steering Committee meeting notes and weigh in by filing an issue or creating a PR against their repo. They have an open meeting on the first Monday at 8am PT of every month. They can also be contacted at their public mailing list steering@kubernetes.io.
You can see what the Steering Committee meetings are all about by watching past meetings on the YouTube Playlist.
If you want to meet some of the newly elected Steering Committee members, join us for the Steering AMA at the Kubernetes Contributor Summit North America 2024 in Salt Lake City.
This post was adapted from one written by the Contributor Comms Subproject. If you want to write stories about the Kubernetes community, learn more about us.
-
Spotlight on CNCF Deaf and Hard-of-hearing Working Group (DHHWG)
In recognition of Deaf Awareness Month and the importance of inclusivity in the tech community, we are spotlighting Catherine Paganini, facilitator and one of the founding members of CNCF Deaf and Hard-of-Hearing Working Group (DHHWG). In this interview, Sandeep Kanabar, a deaf member of the DHHWG and part of the Kubernetes SIG ContribEx Communications team, sits down with Catherine to explore the impact of the DHHWG on cloud native projects like Kubernetes.
Sandeep’s journey is a testament to the power of inclusion. Through his involvement in the DHHWG, he connected with members of the Kubernetes community who encouraged him to join SIG ContribEx - the group responsible for sustaining the Kubernetes contributor experience. In an ecosystem where open-source projects are actively seeking contributors and maintainers, this story highlights how important it is to create pathways for underrepresented groups, including those with disabilities, to contribute their unique perspectives and skills.
In this interview, we delve into Catherine’s journey, the challenges and triumphs of establishing the DHHWG, and the vision for a more inclusive future in cloud native. We invite Kubernetes contributors, maintainers, and community members to reflect on the significance of empathy, advocacy, and community in fostering a truly inclusive environment for all, and to think about how they can support efforts to increase diversity and accessibility within their own projects.
Introduction
Sandeep Kanabar (SK): Hello Catherine, could you please introduce yourself, share your professional background, and explain your connection to the Kubernetes ecosystem?
Catherine Paganini (CP): I'm the Head of Marketing at Buoyant, the creator of Linkerd, the CNCF-graduated service mesh, and 5th CNCF project. Four years ago, I started contributing to open source. The initial motivation was to make cloud native concepts more accessible to newbies and non-technical people. Without a technical background, it was hard for me to understand what Kubernetes, containers, service meshes, etc. mean. All content was targeted at engineers already familiar with foundational concepts. Clearly, I couldn't be the only one struggling with wrapping my head around cloud native.
My first contribution was the CNCF Landscape Guide, which I co-authored with my former colleague Jason Morgan. Next, we started the CNCF Glossary, which explains cloud native concepts in simple terms. Today, the glossary has been (partially) localised into 14 languages!
Currently, I'm the co-chair of the TAG Contributor Strategy and the Facilitator of the Deaf and Hard of Hearing Working Group (DHHWG) and Blind and Visually Impaired WG (BVIWG), which is still in formation. I'm also working on a new Linux Foundation (LF) initiative called ABIDE (Accessibility and Belonging through Inclusion, Diversity, and Equity), so stay tuned to learn more about it!
Motivation and early milestones
SK: That's inspiring! Building on your passion for accessibility, what motivated you to facilitate the creation of the DHHWG? Was there a speecifc moment or experience that sparked this initiative?
CP: Last year at KubeCon Amsterdam, I learned about a great initiative by Jay Tihema that creates pathways for Maori youth into cloud native and open source. While telling my CODA (children of deaf adults) high school friend about it, I thought it'd be great to create something similar for deaf folks. A few months later, I posted about it in a LinkedIn post that the CNCF shared. Deaf people started to reach out, wanting to participate. And the rest is history.
SK: Speaking of history, since its launch, how has the DHHWG evolved? Could you highlight some of the key milestones or achievements the group has reached recently?
CP: Our WG is about a year old. It started with a few deaf engineers and me brainstorming how to make KubeCon more accessible. We published an initial draft of Best practices for an inclusive conference and shared it with the LF events team. KubeCon Chicago was two months later, and we had a couple of deaf attendees. It was the first KubeCon accessible to deaf signers. Destiny, one of our co-chairs, even participated in a keynote panel. It was incredible how quickly everything happened!
DHHWG members at KubeCon Chicago
The team has grown since then, and we've been able to do much more. With a kiosk in the project pavilion, an open space discussion, a sign language crash course, and a few media interviews, KubeCon Paris had a stronger advocacy and outreach focus. Check out this video of our team in Paris to get a glimpse of all the different KubeCon activities — it was such a great event! The team also launched the first CNCF Community Group in sign language, Deaf in Cloud Native, a glossary team that creates sign language videos for each technical term to help standardize technical signs across the globe. It's crazy to think that it all happened within one year!
Overcoming challenges and addressing misconceptions
SK: That's remarkable progress in just a year! Building such momentum must have come with its challenges. What barriers have you encountered in facilitating the DHHWG, and how did you and the group work to overcome them?
CP: The support from the community, LF, and CNCF has been incredible. The fact that we achieved so much is proof of it. The challenges are more in helping some team members overcome their fear of contributing. Most are new to open source, and it can be intimidating to put your work out there for everyone to see. The fear of being criticized in public is real; however, as they will hopefully realize over time, our community is incredibly supportive. Instead of criticizing, people tend to help improve the work, leading to better outcomes.
SK: Are there any misconceptions about the deaf and hard-of-hearing community in tech that you'd like to address?
CP: Deaf and hard of hearing individuals are very diverse — there is no one-size-fits-all. Some deaf people are oral (speak), others sign, while some lip read or prefer captions. It generally depends on how people grew up. While some people come from deaf families and sign language is their native language, others were born into hearing families who may or may not have learned how to sign. Some deaf people grew up surrounded by hearing people, while others grew up deeply embedded in Deaf culture. Hard-of-hearing individuals, on the other hand, typically can communicate well with hearing peers one-on-one in quiet settings, but loud environments or conversations with multiple people can make it hard to follow the conversation. Most rely heavily on captions. Each background and experience will shape their communication style and preferences. In short, what works for one person, doesn't necessarily work for others. So never assume and always ask about accessibility needs and preferences.
Impact and the role of allies
SK: Can you share some key impacts/outcomes of the conference best practices document?
CP: Here are the two most important ones: Captions should be on the monitor, not in an app. That's especially important during technical talks with live demos. Deaf and hard of hearing attendees will miss important information switching between captions on their phone and code on the screen.
Interpreters are most valuable during networking, not in talks (with captions). Most people come to conferences for the hallway track. That is no different for deaf attendees. If they can't network, they are missing out on key professional connections, affecting their career prospects.
SK: In your view, how crucial is the role of allies within the DHHWG, and what contributions have they made to the group’s success?
CP: Deaf and hard of hearing individuals are a minority and can only do so much. Allies are the key to any diversity and inclusion initiative. As a majority, allies can help spread the word and educate their peers, playing a key role in scaling advocacy efforts. They also have the power to demand change. It's easy for companies to ignore minorities, but if the majority demands that their employers be accessible, environmentally conscious, and good citizens, they will ultimately be pushed to adapt to new societal values.
Expanding DEI efforts and future vision
SK: The importance of allies in driving change is clear. Beyond the DHHWG, are you involved in any other DEI groups or initiatives within the tech community?
CP: As mentioned above, I'm working on an initiative called ABIDE, which is still work in progress. I don't want to share too much about it yet, but what I can say is that the DHHWG will be part of it and that we just started a Blind and Visually Impaired WG (BVIWG). ABIDE will start by focusing on accessibility, so if anyone reading this has an idea for another WG, please reach out to me via the CNCF Slack @Catherine Paganini.
SK: What does the future hold for the DHHWG? Can you share details about any ongoing or upcoming initiatives?
CP: I think we've been very successful in terms of visibility and awareness so far. We can't stop, though. Awareness work is ongoing, and most people in our community haven't heard about us or met anyone on our team yet, so a lot of work still lies ahead.
DHHWG members at KubeCon Paris
The next step is to refocus on advocacy. The same thing we did with the conference best practices but for other areas. The goal is to help educate the community about what real accessibility looks like, how projects can be more accessible, and why employers should seriously consider deaf candidates while providing them with the tools they need to conduct successful interviews and employee onboarding. We need to capture all that in documents, publish it, and then get the word out. That last part is certainly the most challenging, but it's also where everyone can get involved.
Call to action
SK: Thank you for sharing your insights, Catherine. As we wrap up, do you have any final thoughts or a call to action for our readers?
CP: As we build our accessibility page, check in regularly to see what's new. Share the docs with your team, employer, and network — anyone, really. The more people understand what accessibility really means and why it matters, the more people will recognize when something isn't accessible, and be able to call out marketing-BS, which, unfortunately, is more often the case than not. We need allies to help push for change. No minority can do this on their own. So please learn about accessibility, keep an eye out for it, and call it out when something isn't accessible. We need your help!
Wrapping up
Catherine and the DHHWG's work exemplify the power of community and advocacy. As we celebrate Deaf Awareness Month, let's reflect on her role as an ally and consider how we can all contribute to building a more inclusive tech community, particularly within open-source projects like Kubernetes.
Together, we can break down barriers, challenge misconceptions, and ensure that everyone feels welcome and valued. By advocating for accessibility, supporting initiatives like the DHHWG, and fostering a culture of empathy, we can create a truly inclusive and welcoming space for all.
-
Spotlight on SIG Scheduling
In this SIG Scheduling spotlight we talked with Kensei Nakada, an approver in SIG Scheduling.
Introductions
Arvind: Hello, thank you for the opportunity to learn more about SIG Scheduling! Would you like to introduce yourself and tell us a bit about your role, and how you got involved with Kubernetes?
Kensei: Hi, thanks for the opportunity! I’m Kensei Nakada (@sanposhiho), a software engineer at Tetrate.io. I have been contributing to Kubernetes in my free time for more than 3 years, and now I’m an approver of SIG Scheduling in Kubernetes. Also, I’m a founder/owner of two SIG subprojects, kube-scheduler-simulator and kube-scheduler-wasm-extension.
About SIG Scheduling
AP: That's awesome! You've been involved with the project since a long time. Can you provide a brief overview of SIG Scheduling and explain its role within the Kubernetes ecosystem?
KN: As the name implies, our responsibility is to enhance scheduling within Kubernetes. Specifically, we develop the components that determine which Node is the best place for each Pod. In Kubernetes, our main focus is on maintaining the kube-scheduler, along with other scheduling-related components as part of our SIG subprojects.
AP: I see, got it! That makes me curious--what recent innovations or developments has SIG Scheduling introduced to Kubernetes scheduling?
KN: From a feature perspective, there have been several enhancements to
PodTopologySpread
recently.PodTopologySpread
is a relatively new feature in the scheduler, and we are still in the process of gathering feedback and making improvements.Most recently, we have been focusing on a new internal enhancement called QueueingHint which aims to enhance scheduling throughput. Throughput is one of our crucial metrics in scheduling. Traditionally, we have primarily focused on optimizing the latency of each scheduling cycle. QueueingHint takes a different approach, optimizing when to retry scheduling, thereby reducing the likelihood of wasting scheduling cycles.
A: That sounds interesting! Are there any other interesting topics or projects you are currently working on within SIG Scheduling?
KN: I’m leading the development of
QueueingHint
which I just shared. Given that it’s a big new challenge for us, we’ve been facing many unexpected challenges, especially around the scalability, and we’re trying to solve each of them to eventually enable it by default.And also, I believe kube-scheduler-wasm-extension (a SIG subproject) that I started last year would be interesting to many people. Kubernetes has various extensions from many components. Traditionally, extensions are provided via webhooks (extender in the scheduler) or Go SDK (Scheduling Framework in the scheduler). However, these come with drawbacks - performance issues with webhooks and the need to rebuild and replace schedulers with Go SDK, posing difficulties for those seeking to extend the scheduler but lacking familiarity with it. The project is trying to introduce a new solution to this general challenge - a WebAssembly based extension. Wasm allows users to build plugins easily, without worrying about recompiling or replacing their scheduler, and sidestepping performance concerns.
Through this project, SIG Scheduling has been learning valuable insights about WebAssembly's interaction with large Kubernetes objects. And I believe the experience that we’re gaining should be useful broadly within the community, beyond SIG Scheduling.
A: Definitely! Now, there are 8 subprojects inside SIG Scheduling. Would you like to talk about them? Are there some interesting contributions by those teams you want to highlight?
KN: Let me pick up three subprojects: Kueue, KWOK and descheduler.
- Kueue
- Recently, many people have been trying to manage batch workloads with Kubernetes, and in 2022, Kubernetes community founded WG-Batch for better support for such batch workloads in Kubernetes. Kueue is a project that takes a crucial role for it. It’s a job queueing controller, deciding when a job should wait, when a job should be admitted to start, and when a job should be preempted. Kueue aims to be installed on a vanilla Kubernetes cluster while cooperating with existing matured controllers (scheduler, cluster-autoscaler, kube-controller-manager, etc).
- KWOK
- KWOK is a component in which you can create a cluster of thousands of Nodes in seconds. It’s mostly useful for simulation/testing as a lightweight cluster, and actually another SIG sub project kube-scheduler-simulator uses KWOK background.
- descheduler
- Descheduler is a component recreating pods that are running on undesired Nodes. In Kubernetes,
scheduling constraints (
PodAffinity
,NodeAffinity
,PodTopologySpread
, etc) are honored only at Pod schedule, but it’s not guaranteed that the contrtaints are kept being satisfied afterwards. Descheduler evicts Pods violating their scheduling constraints (or other undesired conditions) so that they’re recreated and rescheduled. - Descheduling Framework
- One very interesting on-going project, similar to Scheduling Framework in the scheduler, aiming to make descheduling logic extensible and allow maintainers to focus on building a core engine of descheduler.
AP: Thank you for letting us know! And I have to ask, what are some of your favorite things about this SIG?
KN: What I really like about this SIG is how actively engaged everyone is. We come from various companies and industries, bringing diverse perspectives to the table. Instead of these differences causing division, they actually generate a wealth of opinions. Each view is respected, and this makes our discussions both rich and productive.
I really appreciate this collaborative atmosphere, and I believe it has been key to continuously improving our components over the years.
Contributing to SIG Scheduling
AP: Kubernetes is a community-driven project. Any recommendations for new contributors or beginners looking to get involved and contribute to SIG scheduling? Where should they start?
KN: Let me start with a general recommendation for contributing to any SIG: a common approach is to look for good-first-issue. However, you'll soon realize that many people worldwide are trying to contribute to the Kubernetes repository.
I suggest starting by examining the implementation of a component that interests you. If you have any questions about it, ask in the corresponding Slack channel (e.g., #sig-scheduling for the scheduler, #sig-node for kubelet, etc). Once you have a rough understanding of the implementation, look at issues within the SIG (e.g., sig-scheduling), where you'll find more unassigned issues compared to good-first-issue ones. You may also want to filter issues with the kind/cleanup label, which often indicates lower-priority tasks and can be starting points.
Specifically for SIG Scheduling, you should first understand the Scheduling Framework, which is the fundamental architecture of kube-scheduler. Most of the implementation is found in pkg/scheduler. I suggest starting with ScheduleOne function and then exploring deeper from there.
Additionally, apart from the main kubernetes/kubernetes repository, consider looking into sub-projects. These typically have fewer maintainers and offer more opportunities to make a significant impact. Despite being called "sub" projects, many have a large number of users and a considerable impact on the community.
And last but not least, remember contributing to the community isn’t just about code. While I talked a lot about the implementation contribution, there are many ways to contribute, and each one is valuable. One comment to an issue, one feedback to an existing feature, one review comment in PR, one clarification on the documentation; every small contribution helps drive the Kubernetes ecosystem forward.
AP: Those are some pretty useful tips! And if I may ask, how do you assist new contributors in getting started, and what skills are contributors likely to learn by participating in SIG Scheduling?
KN: Our maintainers are available to answer your questions in the #sig-scheduling Slack channel. By participating, you'll gain a deeper understanding of Kubernetes scheduling and have the opportunity to collaborate and network with maintainers from diverse backgrounds. You'll learn not just how to write code, but also how to maintain a large project, design and discuss new features, address bugs, and much more.
Future Directions
AP: What are some Kubernetes-specific challenges in terms of scheduling? Are there any particular pain points?
KN: Scheduling in Kubernetes can be quite challenging because of the diverse needs of different organizations with different business requirements. Supporting all possible use cases in kube-scheduler is impossible. Therefore, extensibility is a key focus for us. A few years ago, we rearchitected kube-scheduler with Scheduling Framework, which offers flexible extensibility for users to implement various scheduling needs through plugins. This allows maintainers to focus on the core scheduling features and the framework runtime.
Another major issue is maintaining sufficient scheduling throughput. Typically, a Kubernetes cluster has only one kube-scheduler, so its throughput directly affects the overall scheduling scalability and, consequently, the cluster's scalability. Although we have an internal performance test (scheduler_perf), unfortunately, we sometimes overlook performance degradation in less common scenarios. It’s difficult as even small changes, which look irrelevant to performance, can lead to degradation.
AP: What are some upcoming goals or initiatives for SIG Scheduling? How do you envision the SIG evolving in the future?
KN: Our primary goal is always to build and maintain extensible and stable scheduling runtime, and I bet this goal will remain unchanged forever.
As already mentioned, extensibility is key to solving the challenge of the diverse needs of scheduling. Rather than trying to support every different use case directly in kube-scheduler, we will continue to focus on enhancing extensibility so that it can accommodate various use cases. kube-scheduler-wasm-extension that I mentioned is also part of this initiative.
Regarding stability, introducing new optimizations like QueueHint is one of our strategies. Additionally, maintaining throughput is also a crucial goal towards the future. We’re planning to enhance our throughput monitoring (ref), so that we can notice degradation as much as possible on our own before releasing. But, realistically, we can't cover every possible scenario. We highly appreciate any attention the community can give to scheduling throughput and encourage feedback and alerts regarding performance issues!
Closing Remarks
AP: Finally, what message would you like to convey to those who are interested in learning more about SIG Scheduling?
KN: Scheduling is one of the most complicated areas in Kubernetes, and you may find it difficult at first. But, as I shared earlier, you can find many opportunities for contributions, and many maintainers are willing to help you understand things. We know your unique perspective and skills are what makes our open source so powerful 😊
Feel free to reach out to us in Slack (#sig-scheduling) or meetings. I hope this article interests everyone and we can see new contributors!
AP: Thank you so much for taking the time to do this! I'm confident that many will find this information invaluable for understanding more about SIG Scheduling and for contributing to the SIG.
-
Kubernetes v1.31: kubeadm v1beta4
As part of the Kubernetes v1.31 release,
kubeadm
is adopting a new (v1beta4) version of its configuration file format. Configuration in the previous v1beta3 format is now formally deprecated, which means it's supported but you should migrate to v1beta4 and stop using the deprecated format. Support for v1beta3 configuration will be removed after a minimum of 3 Kubernetes minor releases.In this article, I'll walk you through key changes; I'll explain about the kubeadm v1beta4 configuration format, and how to migrate from v1beta3 to v1beta4.
You can read the reference for the v1beta4 configuration format: kubeadm Configuration (v1beta4).
A list of changes since v1beta3
This version improves on the v1beta3 format by fixing some minor issues and adding a few new fields.
To put it simply,
- Two new configuration elements: ResetConfiguration and UpgradeConfiguration
- For InitConfiguration and JoinConfiguration,
dryRun
mode andnodeRegistration.imagePullSerial
are supported - For ClusterConfiguration, there are new fields including
certificateValidityPeriod
,caCertificateValidityPeriod
,encryptionAlgorithm
,dns.disabled
andproxy.disabled
. - Support
extraEnvs
for all control plan components extraArgs
changed from a map to structured extra arguments for duplicates- Add a
timeouts
structure for init, join, upgrade and reset.
For details, you can see the official document below:
- Support custom environment variables in control plane components under
ClusterConfiguration
. UseapiServer.extraEnvs
,controllerManager.extraEnvs
,scheduler.extraEnvs
,etcd.local.extraEnvs
. - The ResetConfiguration API type is now supported in v1beta4. Users are able to reset a node by passing
a
--config
file tokubeadm reset
. dryRun
mode is now configurable in InitConfiguration and JoinConfiguration.- Replace the existing string/string extra argument maps with structured extra arguments that support duplicates.
The change applies to
ClusterConfiguration
-apiServer.extraArgs
,controllerManager.extraArgs
,scheduler.extraArgs
,etcd.local.extraArgs
. Also tonodeRegistrationOptions.kubeletExtraArgs
. - Added
ClusterConfiguration.encryptionAlgorithm
that can be used to set the asymmetric encryption algorithm used for this cluster's keys and certificates. Can be one of "RSA-2048" (default), "RSA-3072", "RSA-4096" or "ECDSA-P256". - Added
ClusterConfiguration.dns.disabled
andClusterConfiguration.proxy.disabled
that can be used to disable the CoreDNS and kube-proxy addons during cluster initialization. Skipping the related addons phases, during cluster creation will set the same fields totrue
. - Added the
nodeRegistration.imagePullSerial
field inInitConfiguration
andJoinConfiguration
, which can be used to control if kubeadm pulls images serially or in parallel. - The UpgradeConfiguration kubeadm API is now supported in v1beta4 when passing
--config
tokubeadm upgrade
subcommands. For upgrade subcommands, the usage of component configuration for kubelet and kube-proxy, as well as InitConfiguration and ClusterConfiguration, is now deprecated and will be ignored when passing--config
. - Added a
timeouts
structure toInitConfiguration
,JoinConfiguration
,ResetConfiguration
andUpgradeConfiguration
that can be used to configure various timeouts. TheClusterConfiguration.timeoutForControlPlane
field is replaced bytimeouts.controlPlaneComponentHealthCheck
. TheJoinConfiguration.discovery.timeout
is replaced bytimeouts.discovery
. - Added a
certificateValidityPeriod
andcaCertificateValidityPeriod
fields toClusterConfiguration
. These fields can be used to control the validity period of certificates generated by kubeadm during sub-commands such asinit
,join
,upgrade
andcerts
. Default values continue to be 1 year for non-CA certificates and 10 years for CA certificates. Also note that only non-CA certificates are renewable bykubeadm certs renew
.
These changes simplify the configuration of tools that use kubeadm and improve the extensibility of kubeadm itself.
How to migrate v1beta3 configuration to v1beta4?
If your configuration is not using the latest version, it is recommended that you migrate using the kubeadm config migrate command.
This command reads an existing configuration file that uses the old format, and writes a new file that uses the current format.
Example
Using kubeadm v1.31, run
kubeadm config migrate --old-config old-v1beta3.yaml --new-config new-v1beta4.yaml
How do I get involved?
Huge thanks to all the contributors who helped with the design, implementation, and review of this feature:
- Lubomir I. Ivanov (neolit123)
- Dave Chen(chendave)
- Paco Xu (pacoxu)
- Sata Qiu(sataqiu)
- Baofa Fan(carlory)
- Calvin Chen(calvin0327)
- Ruquan Zhao(ruquanzhao)
For those interested in getting involved in future discussions on kubeadm configuration, you can reach out kubeadm or SIG-cluster-lifecycle by several means:
- v1beta4 related items are tracked in kubeadm issue #2890.
- Slack: #kubeadm or #sig-cluster-lifecycle
- Mailing list